Cloud cost management has become a weekly ritual nobody enjoys.
Open Cost Explorer. Check GCP Billing exports. Review Azure consumption reports.
Try to explain — again — where that cost spike came from.
The industry keeps selling « visibility » as the answer. More dashboards, more alerts, more reports.
But dashboards don’t prevent waste. They observe it. Often too late to matter.
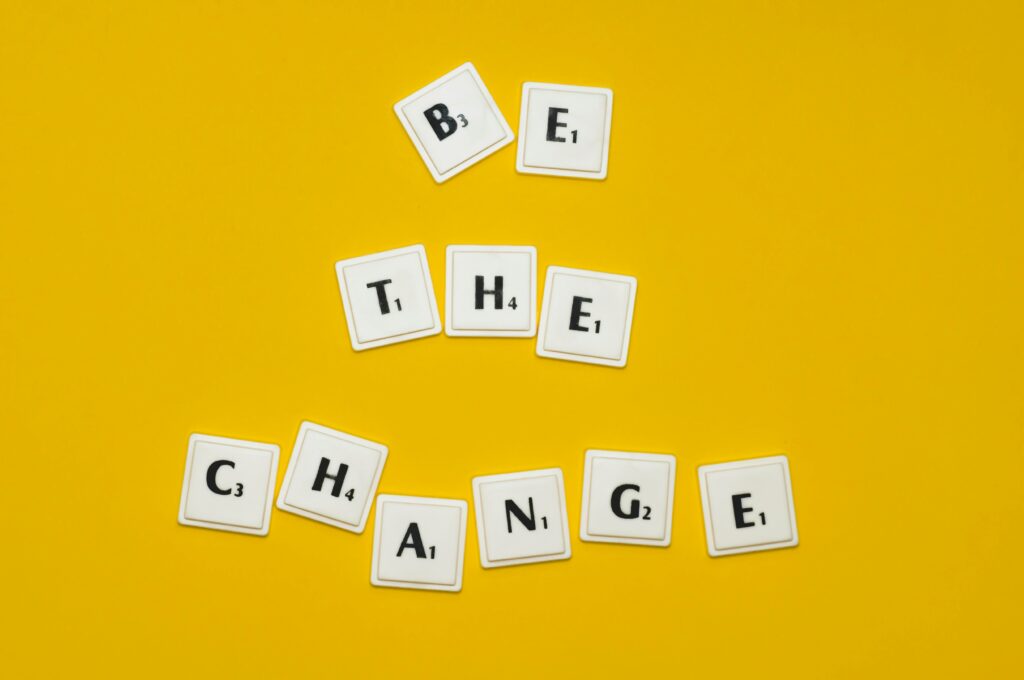
Why the Current FinOps SaaS Model Fails
Most FinOps SaaS tools work the same way: they aggregate your data, generate reports, and charge a percentage of your cloud spend — often 1 to 3%. The more you spend, the more they earn.
There’s something fundamentally broken in paying a markup on your own waste while trying to reduce it. That’s not alignment — that’s just another middleman extracting rent.
These tools rarely solve the real problem. At best, they show you where the budget leaked. At worst, they bury your team under dashboards and alerts no one has time to interpret.
The Real Bottleneck: Engineers Doing the Wrong Work
The true bottleneck in FinOps isn’t data — it’s time.
Highly-skilled engineers end up stuck in reactive loops:
- Manually hunting down idle resources
- Retagging services for reporting accuracy
- Writing post-mortems instead of preventing issues upstream
This is not value-adding work. It’s operational debt. And it scales badly.
FinOps, in most teams, becomes a side-task — a burden inherited by the staff engineer or the lead DevOps — not a function designed for continuous improvement.
A Better Path: Contextual AI Agents that Assist, Not Automate Blindly
The future isn’t about full automation. It’s about contextual intelligence that helps engineers refocus on what they’re good at: building and optimizing systems.
A good FinOps agent doesn’t trigger rule-based automations. It connects the dots — usage, cost, tags, ownership — and surfaces insights when they’re actionable.
The value isn’t in acting for you, but in saving you the investigation time and providing just enough context to make the decision obvious.
Example 1 — Digest Generation: Turning Monthly Reporting from Pain to Insight
Every tech team knows the end-of-month reporting ritual: gathering cost data, investigating why RDS or S3 spiked, and trying to explain it to finance.
The agent changes the dynamic. Instead of dashboards or manual queries, it compiles a monthly digest like:
Copied!Monthly Digest - March Recap for Team Infra ✅ RDS costs increased by +$3.4K (+18%) Context: - Linked to the new read replica added on March 4th. - Replica was necessary due to API latency issues. ✅ S3 costs down by -$2.1K (-12%) - Cleanup script executed on 'data-archive-2021' bucket. - 3.4TB of old snapshots deleted. Open questions: - Kubernetes cluster 'batch-processing' idle 40% of the time. Estimated $5K/month impact. → Do we plan to right-size next sprint? Click to open the detailed view or snooze this reminder.
Instead of spending days re-constructing the story, the team receives a structured summary, focused on what matters, ready to share.
Example 2 — Anomaly Investigation: Proactively Surfacing What Needs Attention
Take the classic scenario: S3 storage costs slowly creeping up — unnoticed until the end of the month when someone finally checks.
A contextual FinOps agent changes the dynamic. Instead of relying on someone noticing, it proactively sends this:
Copied!Hi Janet, We detected a change in cost trend on 'video-prod' starting May 11. - Historical: costs stable at $18K/month for the past 3 months - Since Nov 11: upward trend of +15% month-over-month - Projected annual impact if sustained: +$30K/year Context: - Similar behavior observed last quarter due to crawler activity - Storage Class: Standard, no recent lifecycle policy changes detected Suggested next steps: [1] Create a GitHub issue for investigation [2] Notify @video_team [3] Snooze for 7 days and re-check
The agent isn’t automating a fix — it’s surfacing structured context fast, connecting usage patterns, historical behavior, and potential causes.
The team doesn’t waste hours digging through metrics — they start from insight and decide what to do next.
Why Context Beats Rule-Based Automation
The cloud is too dynamic for static rules. What looks wasteful one day may be necessary the next. Context is what makes the difference.
A contextual agent understands:
- Service and team ownership
- Historical usage patterns
- Real architectural intent, not just cost deltas
It doesn’t ping you for every threshold breach. It tells you why something’s unusual — and whether it’s worth your attention.
The goal isn’t to automate termination scripts. It’s to ensure engineers don’t waste time rediscovering what the system already knows.
Shifting FinOps Left — Back Into Engineering Hands
With this model, FinOps stops being a centralized, finance-led process and becomes part of the engineering loop.
- Engineers get cost context directly in their workflows — monthly, weekly, or on-demand
- Infra leads stop wasting cycles reconciling CUR exports
- Product knows the trade-offs of an experiment before it hits production budgets
FinOps shifts from reactive reporting to proactive decision-making — owned by engineering, where it belongs.
Where It Ends: No More “FinOps Dashboards”
The outcome is clear.
No more dashboards full of cost center breakdowns nobody reads.
No more engineers wasting cycles explaining where the money went — long after it’s gone.
No more « visibility tools » profiting as your bill grows.
Instead, you get a system that quietly works in the background, surfaces context when it matters, and gets out of the way when it doesn’t.
Engineers stay focused on what they’re supposed to do: building, optimizing, and running systems — not babysitting dashboards.
Final Thoughts
FinOps isn’t a role. It’s not a team.
It’s a capability that should be embedded into the engineering loop — and an AI agent is how we get there.
The best FinOps interface isn’t a dashboard.
It’s no interface at all — just enough context, at the right moment, to help engineers make better calls.